This article explores the role of knowledge graphs in data fabrics. If you want to learn more about this topic, please join Forrester Analyst Noel Yuhanna and Cambridge Semantics Co-Founder Ben Szekely at an interactive discussion on this topic. Learn more here.
— — — — — — — —
Sometimes in hindsight the “killer use case” for a new technology or idea seems obvious as though the inventor knew from day one what to do with her new idea. But just as often finding the ideal application for a novel invention can take a while. Take modern trains for example. By 1900, trains had all but replaced canals as the dominant means of commercial transportation, but in the early days when modern trains were just catching on, some people believed they would complement rather than displacing canals by replacing the mules that towed the boats. While foolish in retrospect, at the time this idea probably made sense.
Today a good example of a new idea that has been around for awhile, but which is just now finding its killer use case is the use of knowledge graphs as part of a data fabric architecture.
First defined in the 1970s, knowledge graphs are now part of our daily lives with digital platforms like Google, LinkedIn, and Facebook using them to create linked datasets which we experience as search results, professional connections, or suggested friends.
“A Knowledge Graph is a connected graph of data and associated metadata applied to model, integrate and access an organization’s information assets. The knowledge graph represents real-world entities, facts, concepts, and events as well as all the relationships between them yielding a more accurate and more comprehensive representation of an organization’s data.”
Data fabrics, in contrast, are a newer idea, first formally defined in 2016 by Forrester Analyst Noel Yuhanna, in his report “Big Data Fabric Drives Innovation and Growth.” A data fabric is a modern data management architecture that allows organizations to use more of their data more often and more quickly to fuel analytics, digital transformation, and other high stakes business processes. It enables these advantages by proactively preparing, integrating, and modeling data from numerous, diverse, enterprise source systems into an integrated platform of business-ready data for on-demand access. Forrester defines a data fabric as:
“A Data Fabric is (a data management architecture that) orchestrates disparate data sources intelligently and securely in a self-service and automated manner, leveraging data platforms such as data lakes, Hadoop, Spark, in-memory, data warehouse, and NoSQL to deliver a unified, trusted, and comprehensive real-time view of customer and business data across the enterprise.”
Source: Enterprise Data Fabric Enables DataOpAdvanced Level: Data Practices For Insights-Driven Businesses, 12.23.20
Within a data fabric architecture, a knowledge graph brings much needed capabilities in several key areas. They connect related data across silos with enormous flexibility, linking thousands or millions of related points of data from across the business and bringing unprecedented granularity and flexibility in the data integration process. (Learn how this works.) Knowledge graphs also make complicated data much easier to understand and use, by establishing a semantic layer of business definitions and terms on top of the often cryptic and highly technical names assigned to individual fields of data at the schema or application layer.
By allowing organizations to excavate data from often large repositories of unstructured files (think documents, emails, chats, or PDFs), knowledge graphs allow organizations to tap into more of their enterprise data reserves to fuel analytics. And finally, knowledge graphs make the overall data fabric more flexible and easier to build out incrementally over time, thus lowering risk and speeding deployment. They do this by allowing the organization to build the fabric in stages — starting with one data domain, or high value use case, and building that into the initial knowledge graph model, and then incrementally expanding that over time with more data, user cases, and users.

What it Takes
To deliver these capabilities, the knowledge graph layer within the data fabric requires some specific attributes.
Any Data in Any Format
First it must integrate data from any and all enterprise source platforms, regardless of the sources’ structure variation, format differences, respective data models, or other distinctions at origination. This includes common structured sources like relational databases and flat files, as well as semi structured data like JSON or XML files and unstructured sources like PDFs or documents.
Performant Loading and Efficient Storage
By nature a knowledge graph is (or at least has the chops to become) an enterprise-scale platform. This is impossible to achieve without automating — and expediting — loading source data into the graph and providing options for efficient storing of graph data. In the context of the data fabric, this means ensuring that the knowledge graph provides options on how data from sources is connected into the graph, from full on data replication and onboarding, to data on demand and virtualization (quick tutorial on virtualization).
Flexible Deployment
It’s important that the organization implementing the data fabric and knowledge graph have the option to deploy it wherever they want including on-premises, in the cloud or in a hybrid model. The most affordable operational environments typically do not require additional investments. The knowledge graph platform should give users the flexibility to leverage commodity VMs on-premises and in any cloud type applying standard deployment mechanisms like Kubernetes. Clouds deployment options include, for example, on-premise hybrids, public or private options, or public-private hybrids. (More on data fabrics in the cloud.)
Interactive Query at Scale
The ultimate goal of a data fabric is to produce a vast number of analytic-ready blended datasets very quickly to meet the unique and emergent needs of data consumers across the organization. To make this possible the knowledge graph must handle a large number of queries quickly, even when those queries involve pulling data from far flung corners of very large graph models. Fast response times are key, as is the ability to process queries for known questions, as well as unanticipated exploratory queries. Knowledge graphs that use high performance architectures like MPP models and in-memory query execution are best suited to meet this need.
Integrate Easily with Other Components of the Fabric
A data fabric isn’t one product or technology. Rather it’s an architecture that includes many components, some of which are new to the organization while others that are long term incumbents. To work in this context, knowledge graphs need to support open standards that enable all the data, models, and metadata that make up the knowledge graph to be easily synchronized with or exported to other applications.
Enterprise Grade Security and Governance
A large part of readying knowledge graphs for use in a data fabric pertains to fundamentals of security, data governance, and regulatory compliance. Fine-grained access controls are required that specify which users can access, query, and update which parts of the graph and how they can use them. This granular security has significant data governance implications. It reinforces role-based access control for data privacy, while its applicability to PII is perfect for regulatory compliance.
Where to Begin
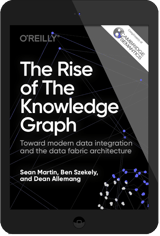
Thought leaders like Etisham Zaidi at the Gartner Group (Recent Gartner Top Ten Data and Analytic Trends for 2021 Report: Graph Connects Everything) and Noel Yuhanna at Forrester Research strongly recommend organizations include a knowledge graph in any data fabric stack. But for ordinary IT and data leaders, often the more pragmatic questions are: “How do I get started?” ”What is the best use case?” “What skills will I need?”and perhaps most vexing, “How do I build a graph representation of my data, something that we have never done before?” Our advice to people who raise these questions to our team at Cambridge Semantics is think big, start small, scale fast, and just get going. An initial 8–10 week project that focuses on a specific use case and a finite set of data sources delivers an initial working knowledge graph, accelerates the learning curve, and gives the team hands-on exposure to the new technology. That first project generally includes more than a few “ah-ha” moments as the team gets its head around how semantic and graph data models work and discovers how its existing skill sets are easily adapted to this new approach.